Artificial intelligence, a powerful tool to combat antimicrobial resistance: An update
Abstract
Antimicrobial resistance (AMR), also known as antibiotic resistance, is a global health crisis. Many people die from diseases caused by multi-drug resistant (MDR) pathogens. Artificial intelligence (AI) involves creating computer systems that can perform tasks typically requiring human intelligence, such as visual perception, speech recognition, decision-making, and language translation. AI has been successfully used in various fields, from single-cell study to space science. There is huge potential for using AI in medical science, including fighting against MDR to combat AMR. In this review, we have highlighted some of AI’s successful uses and potentiality to combat AMR for the betterment of the human race, including the basic principles of AI, available AI resources, use and scope, advantages and limitations. In addition, in this review, we also have highlighted new research areas such as AMR detection, new drug development etc. to control of AMR.
INTRODUCTION
Antimicrobial resistance (AMR) is when microorganisms become resistant to antimicrobial agents, making them ineffective at inhibiting or killing the microorganisms. Antimicrobials are used to fight against microbes. Bacteria, viruses, fungi, and parasites have developed this resistance over time by modifying their body physiology, genetic structures, and other cellular components and activities because of the selection pressure induced by antimicrobials. After penicillin was introduced to the market in 1941, the first signs of resistance appeared in staphylococci, streptococci, and gonococci. By 1942, Staphylococcus aureus, which was penicillin-resistant, emerged [1].
The World Health Organization (WHO) identifies AMR as one of the top 10 global health threats, making it one of the most concerning phenomena of the 21st century [2]. Up to 10 million deaths could occur each year by 2050. AMR, if unchecked, could lower GDP by US$3.4 trillion yearly and increase the number of people living in extreme poverty by 24 million during the following ten years [3]. A conservative estimate by the Centers for Disease Control and Prevention (CDC) suggests that over two million Americans contract antibiotic-resistant infections annually, with at least 23,000 resulting in death. According to the CDC, AMR costs the United States $55 billion annually, of which $20 billion is spent on direct healthcare costs and $35 billion on societal costs associated with lost productivity [4]. AMR also has a negative impact on achieving Sustainable Developmental Goals (SDG).
Any change in genetic material e.g., DNA or RNA sequence of an organism is known as mutation. Mutation is directly linked with the development of AMR. Mutation due to selection pressure or as a consequence of natural phenomenon e.g., spontaneous plays major role in the development of AMR. These mutations ultimately may cause changes in amino acid sequence of protein such as receptor proteins, and enzymes that are potential target of many antibiotics. Once altered antibiotics are not able to interact with receptor protein, binding protein, target enzymes resulting resistance development.
Selective pressure can also drive the development of AMR by promoting the survival and proliferation of organisms with novel mutations or newly developed characteristics [5]. Factors including overuse and inappropriate use (inappropriate choices, inadequate dosing, and poor adherence to treatment guidelines) contribute to developing antibiotic resistance [6]. Dever et al. [7] claim that there are primarily three primary mechanisms by which AMR developed: alterations in membrane permeability to antibiotics, transformation of bacterial proteins that are antimicrobial targets, and enzymatic degradation of antibacterial agents. It can be kept on the bacterial chromosome or by plasmid maintenance [7]. Statistics show that AMR and its effects are gradually growing, and in contrast, the world has produced very few antibiotics. The last new class of antibiotics to be discovered and commercialized was fluoroquinolones, the previous group of broad-spectrum antibiotics in 1987. It is, therefore, high time to put all our efforts into using an integrated approach to develop new antimicrobials, methods, techniques, and strategies to work against AMR.
Artificial intelligence (AI), a branch of computer science that deals with creating intelligent systems capable of learning from data and making informed decisions, is increasingly being applied to various aspects of AMR management [8]. Machine learning algorithms, a subset of AI, can analyze vast datasets of microbial genetic information, clinical records, and drug interactions to identify patterns and predict the emergence of antibiotic-resistant strains. These algorithms can help healthcare professionals make more informed decisions about antibiotic use, ensuring that treatments are both effective and tailored to the specific microorganisms involved. AI-powered diagnostics are revolutionizing the identification of infectious agents and their susceptibility to antibiotics. Rapid and accurate diagnosis is crucial for effective treatment and reducing the unnecessary use of antibiotics. AI-driven diagnostic tools can quickly analyze biological samples and provide insights into the most suitable treatment options [9]. In addition to diagnostics, AI is playing a pivotal role in drug discovery. Traditional drug discovery processes are time-consuming and costly, but AI algorithms can sift through vast chemical libraries and predict potential antimicrobial compounds with higher efficiency. This accelerates the development of new antibiotics and alternative treatments, which is essential given the stagnant pace of antibiotic discovery in recent decades [10, 11]. Moreover, AI-powered surveillance systems continuously monitor antimicrobial usage, resistance patterns, and disease outbreaks [12]. By analyzing this real-time data, AI can help identify regions or facilities with elevated AMR risks, enabling targeted interventions to prevent its spread.
As we confront the escalating threat of AMR, the integration of AI technologies into our strategies for research, diagnosis, and treatment represents a promising avenue. With AI's capacity to process and analyze data at unprecedented speeds, it offers hope in the battle against AMR and the pursuit of innovative solutions to protect public health and achieve (SDGs). Collaborative efforts that harness the power of AI alongside traditional approaches will be crucial in addressing this 21st-century global health challenge. The aim of this review is therefore to update our current knowledge on the basic principles of AI along with areas having potential to apply AI for combating AMR.
AMR IN HUMAN HEALTH
In this running era, AMR mainly affects human life in several ways, as humans are the ultimate consumers of animal products and have a close relationship with the environment. The most pressing risks to public health pose substantial obstacles to the effective prevention and treatment of chronic diseases and raise the expense of healthcare [13]. AMR is thought to cost the European Union more than nine billion euros annually [14]. Moreover, antibiotic resistance increases healthcare costs by $20 billion annually and causes an estimated $35 billion in lost productivity according to the CDC, in the United States [15].
According to the statistical model developed by Murray et al., [16] bacterial AMR will be directly responsible for 12.7 million of the estimated 495 million deaths in 2019. Regionally, the all-age mortality rate caused by resistance was highest in western sub-Saharan Africa, at 27.3, and lowest in Australasia, at 65. Around 1.5 million deaths in 2019 were caused by resistance from lower respiratory infections. The six most common pathogens for mortality associated with resistance caused 929000 deaths related to AMR and 3.57 million deaths linked with AMR in 2019 (Escherichia coli, followed by Staphylococcus aureus, Klebsiella pneumoniae, Streptococcus pneumoniae, Acinetobacter baumannii, and Pseudomonas aeruginosa [16].
A report from 2021 showed that , 35.0% of hospitalized patients in Europe received antibiotics [17]. This number is around 258.0 million in the USA in 2010 with macrolides and penicillin as the most prescribed antibiotics. A report in 2017 from India showed that more than 70% of isolates of Klebsiella pneumoniae, E. coli, and Acinetobacter baumannii as resistant to fluoroquinolones and third-generation cephalosporins [18]. Many of these pathogens are zoonotic in nature. Anthropogenic Actions are believed to be involved in the emerging and re-emerging many zoonotic diseases including spread of AMR [19].
AMR in humans can be caused by several factors, including poor diagnostic tools, self-medication, and unneeded, insufficient, and inefficient doses. South Europe was shown to have a greater rate of self-medication with antibiotics (19%) compared to Northern Europe (3%) and Central Europe (1%). In African nations, all antibiotic use is done without a prescription, compared [20]. Not only does direct human consumption of antibiotics for treatment purposes cause antibiotic resistance but also some indirect factors are responsible for the development of AMR in humans. The use of antibiotics in livestock production is increasing. It has already surpassed the total mass consumed by humans [18]. Because humans consume more animal products than other species, transmission occurs through this pathway. Several models have been proposed to describe the transmission of antimicrobial-resistant bacteria (ARB) and antimicrobial resistance genes (ARGs) between humans and animals. These transmission pathways can occur bidirectionally, with clonal transfer of ARB and horizontal transmission of ARGs being two of the primary conceptual models [21].
AMR IN ANIMALS
Animal production is expanding to supply the demand for protein as the global population grows every day. Antimicrobials are frequently used for four different purposes: 1. Therapeutic, mainly for treating a single animal or a group of animals; 2. Metaphylactic, both for prophylaxis and therapy; 3. prophylactic, for preventing disease; and 4. Subtherapeutic, for promoting growth, improving feed efficiency, and treating disease [22]. Two-thirds of global annual antibiotic production, or 65,000 tons out of 100,000, are utilized for animal husbandry, as per The State of the World's Antibiotics 2015 report [23].
It is estimated that 80% of the antimicrobials consumed annually in the United States are used on animals raised for food [24]. It causes AMR in animals and enters humans via the food chain. Research indicates 228 countries where antibiotics are used in livestock, with a conservative estimate of the total consumption in 2010 being 63,151 tons. By 2030, antimicrobial usage in Brazil, Russia, India, China, and South Africa is projected to increase by 67% [25].
There are several resistant bacteria that have been isolated against different antibiotics in recent time. This indicates the alarming situation of the AMR era in the animals. All Campylobacter isolates from animals, including cattle, chickens, turkeys, pigs, sheep, dogs, cats, and horses, have shown resistance to one or more antimicrobial substance(s), including quinolones, macrolides, lincosamides, chloramphenicol, aminoglycosides, tetracycline, β-lactams, cotrimoxazole, and tylosin [26, 27].
Salmonella spp. also exhibits the same resistance to tetracyclines, sulfonamides, streptomycin, kanamycin, chloramphenicol, β-lactams, amoxicillin/clavulanic acid, nalidixic acid, and ceftriaxone in isolates obtained from humans, chicken, turkey, pig, cats, dogs, and horses [28].
AMR IN ENVIRONMENT
The environment is one of the critical components of One Health. Human and animal populations are always interconnected. Due to rapid and unauthorized as well as without proper maintenance of the withdrawal period of antibiotics in humans and animals, the residue of antibiotics and the antibiotic resistance gene are frequently found in the environment, e.g., soil, air, and water. According to a United Nation Environmental Program (UNEP) assessment, human exposure to AMR bacteria in the environment can happen through contact with contaminated food, drinking water, fungal spores, and other channels [29]. The 2015 Action Plan for AMR developed by the WHO has increasingly emphasized the environment over the past decade.
Both humans and animals can mainly get AMR through food-producing environments, which plays a vital role in spreading AMR. AMR bacteria from various environmental sources, such as crop production and horticulture, can contaminate environments where food is produced. The sources of pollution include waste products like slurry, manure, and air that are produced by animals used for food production on land and in water. Additionally, waste products and leftovers from post-harvest food processing plants (such as slaughterhouses and food processing facilities), urban and hospital wastewater treatment plants, and effluents (resulting from the direct use of antimicrobials) all contribute to environmental pollution [30].
The biologically active form of a significant portion of the antibiotics people eat is eliminated in the urine and faeces. The antibiotics that are often less water soluble, such as norfloxacin, ofloxacin, ciprofloxacin, trimethoprim, sulfamethoxazole, and doxycycline, are those that are more usually discovered in sludge [31]. Compounds ultimately have one of three outcomes when they enter a wastewater treatment facility: biodegradation, absorption into sewage sludge, or unmodified discharge into the effluent. Finally, unaltered antibiotics are responsible for antibiotic resistance [32].
Genes responsible for AMR have been found in different environmental samples in several research worldwide. In the inlet to the treatment plant, there were three types of water: domestic water, domestic waste with hospital effluent, and hospital effluent. After analysis, the sample showed that the resistance of E. coli to third-generation cephalosporin was 25%, 70%, and 95% [33].
Many AMR genes have also been detected in microplastics found in the aquatic environment and have been shown to provide new platforms for biofilm development, which causes different health problems for humans and animals [34]. Besides this, it helps the potential effect of ARGs. ARGs spread easily when manure is distributed widely, by feedlots or land spreading. Antibiotics were found downwind of feedlots, comparable to levels downstream of sewage outlets (0.5-4.6 g/g) [35]. In groundwater, sulfamethazine and chlortetracycline inhibit nitrate growth and denitrification, increasing nitrate pollution risk [36].
ARGs has been found everywhere in the environment, including water, air, and soil, as the lives on the earth greatly depend on the environment and its resources. Hence, taking proper steps to combat the spreading of ARGs through the atmosphere is necessary.
ARTIFICIAL INTELLIGENCE
AI is an alternate way to do specific tasks that work by combining a set of established data and algorithms and doing trained software simultaneously to learn automatically from patterns. There are several definitions of AI: The Turing test is possibly the most widely used definition of AI.; Allan Turing devised an experiment in 1950 called an "imitation game." AI can be described as the ability of a computer to pass the Turing test, a game involving three participants: a human judge, an individual, and an automated system. The participants are separated and can only communicate through text. If the human judge is unable to distinguish between the machine and person, the Turing test is considered a success [37].
"AI" also refers to the science and engineering of creating intelligent machines, and "intelligence" refers to the computational component of the capacity to do tasks in the real world [38]. Another definition of AI is A study area in computer science. AI strives to build tools with human-like learning, reasoning, and self-correction capabilities [39].
When defining AI, several components should include inclusiveness, precision, comprehensiveness, practicability, and permanence [40]. Due to the quick advances, definitions have also evolved throughout time. The phrase "imitating intelligent human conduct," used in more recent reports, is already significantly stronger. Although there are various definitions, most fall into one of the following four categories [39]: 1. systems with human-like thinking, 2. systems that behave like people, 3. systems capable of logical thought, 4. systems that function logically. The fields of AI are Machine learning, Deep learning, and neural networks with different types: Reactive AI, Limited memory machines, Theory of mind, and Self-aware AI.
AI aims to develop software that can provide an explanation based on output and reason based on input. AI will enable human-like interactions with software and provide decision support for specific tasks, but it has yet to be and will be a replacement for people.
AI AND HEALTH SECTOR
AI is crucial in enhancing the health industry by collecting patient data, early diagnosis and prevention of illness, using X-ray, Computerized Tomography (CT), and Magnetic Resonance Imaging (MRI), reducing cost reduction, and improving medication prescribing. For instance, advances in AI are among the most developed tools in this field and have demonstrated great accuracy in imaging and signal identification tasks [41]. AI-based technology will improve life by making it simpler, safer, and more productive. AI has the potential to enhance patient care and reduce healthcare expenses significantly [42]. In many sectors, the manual health system has already been digitally turned into an automated one by AI. In other applications, individuals are no longer required to manage more fundamental medical practice responsibilities like managing patients and medical supplies [43]. They are predicting the medical need, automatic radiation analysis, ophthalmology, robotic surgery, reducing AMR, biofilm detection including beta-lactamase inhibitors, AMR marking, new drug discovery, and safe caring of the patient.
There are some advantages and limitations to AI applications. The usages of AI in different health sectors are briefly presented in Figure 1. Benefits typically assist with early automated decision-making, early diagnosis, excellent systematic work, data exchange, time savings, and performance enhancement. Challenges are areas for improvement in decisions that can be provided s, such as lack of skilled personnel in the health sector to operate AI., patient safety, leaking of patient data, medicine prescribing error, and human interference.
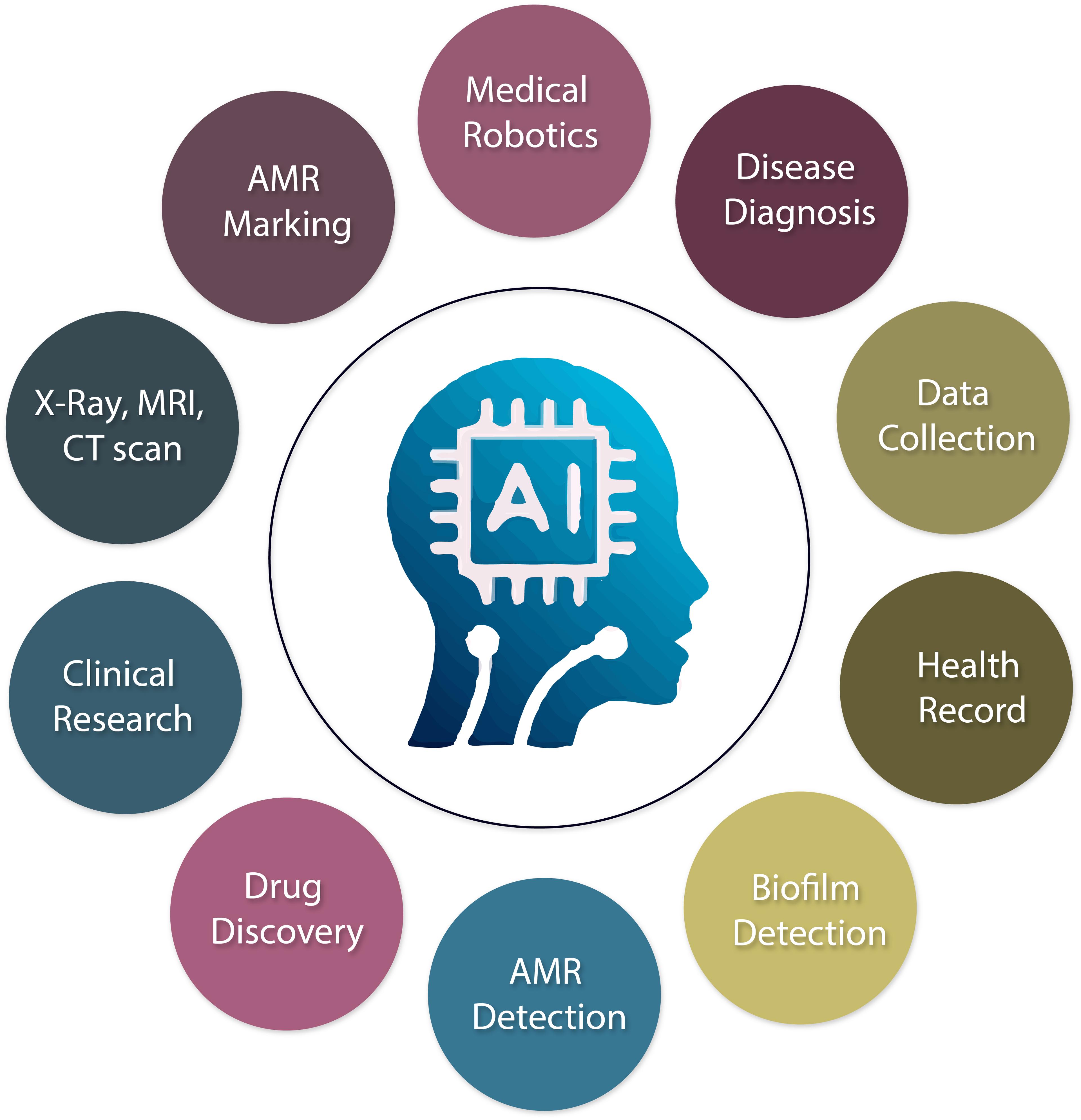
AI AND DISEASE DIAGNOSIS
Identification of the causal agent is required for accurate disease diagnosis, which is crucial for planning, efficient treatment, and ensuring the well-being of patients. Due to the lack of proper diagnosis facilities for infectious or non-infectious diseases and if the condition is infectious, then identifying the causal agent, either by bacteria or viruses, or other organisms, the practitioners prescribe broad-spectrum antibiotics every year, thus creating AMR. The Centers for Disease Control and Prevention (CDC) estimates that around 1.7 million diseases connected to healthcare are treated annually in hospitals in the United States of America. Infections contracted in hospitals are the primary cause of death from infectious diseases and are among the top 10 reasons worldwide. These infections are responsible for around 99,000 deaths each year [44]. Broad-spectrum antibiotics are occasionally administered as a form of treatment without a definitive diagnosis and an indication for antibiotic therapy. Occasionally, a patient's demand for immediate recovery from their illness leads to the serial administration of antibiotics [6, 45].
The disease diagnosis process has become more accessible, quicker, and more accurate due to the advancement of science. For disease diagnosis, AI methods ranging from machine learning to deep learning are becoming increasingly common in the healthcare industry (Table 1). This assists medical professionals in more precisely selecting the appropriate treatments to prescribe. Skin disorders have been found by analyzing primary tumor data from the Institute of Oncology with Multi-Layer Perceptron (MLP) and Artificial Neural Networks [46]. The Urology disease-related heterogeneous dataset used machine learning to identify the urological disease, and 71.8% of the participants concluded that AI is more effective than human intelligence in diagnosing urology disease detection [47]. Gastrointestinal disease Data was collected from humans through IoT Visual Geometry Group (VGG) 16, Artificial Neural Networks (ANN), and Deep Learning Accuracy with an accuracy of 98.4% [48]. Throughout the COVID-19 pandemic, a variety of AI-based diagnostic tools have been utilized [49] to analyze a CT scan dataset by AI and found that 90.9% sensitivity and 87.5% specificity; on the other hand, Chest X-ray dataset by using deep learning models got the accuracy 98.8% [50] and Deep learning and the Health Technology Assessment were used to analyses the RT-PCR laboratory dataset [51]. Deep Learning and Residual Neural Network (ResNet) were used to analyze a sample of one hundred computed tomography (CT) images of tuberculosis [52], which got 85.29% accuracy. Not only that, in recent years, different chronic diseases, different types of cancer, cardiovascular diseases, thyroid disease, and Alzheimer’s disease have been successfully diagnosed using AI.
Deep learning strategies based on AI have been effectively merged with microfluidics to detect bacteria and viruses. Additionally, deep learning has successfully identified antibacterial molecules with bactericidal activity against various pathogens. Because human-operated microscopy is a time-consuming and laborious process with poor precision, researchers can discover and monitor infections for clinical diagnosis and pathogenesis studies using microscopic imaging. This enables the researchers to better understand the disease process [53].
Table 1. Current accessible image collection, utilized in deep learning experiments to identify various microorganisms, aids in precise disease diagnosis.
DISCOVERY AND IDENTIFICATION OF BETA-LACTAMASE INHIBITORS USING AI
For the past 60 years, beta-lactams (including penicillin, cephalosporins, carbapenems, and monobactams) have been the most popular and efficient antibiotics, making up 65 %of all antibiotic usage globally. Additionally, they are among the best treatments for infectious disorders [58].
Antibiotic-resistant bacteria have developed various defenses against therapeutic agents. Antibiotic resistance mechanisms include enzymatic degradation or modification of antibiotics, alteration of the antibiotic's target site, and prevention of access to the target site by altering permeability or efflux. Furthermore, bacteria have enhanced their ability to target antibiotics [59].
One of the main resistance mechanisms is the production of beta-lactamase, a bacterial enzyme that confers multi-resistance to beta-lactam antibiotics like penicillin, cephalosporins, cephamycin, monobactams, and carbapenems. By forming a covalent bond with and inactivating enzymes, -lactam antibiotics prevent the formation and modification of the bacterial peptidoglycan.
There are two structural types of β-lactamases named serine β-lactamases and metallo-β-lactamases (MBL). The serine β-lactamases hydrolyse later-generation cephalosporins, and carbapenemases such as Klebsiella pneumoniae carbapenemases (KPC) that hydrolyse carbapenem antibiotics, in addition to later-generation cephalosporins. Metallo-β-lactamases (MBL) are Zn (II) dependent enzymes that can accommodate most β-lactams in their active site and will hydrolyse almost all β-lactam antibiotics, including carbapenems [60].
Reports are available suggesting that in many cases the available inhibitors do not inhibit many β-lactamases at the moment [61]. In recent years, AI has been used to develop new β-lactamase inhibitors (Figure 2) to combat AMR and the organism. This process uses a Multichannel deep neural network (DeepBLI), Machine learning algorithms, DeepBL Deep Learning-Assisted Photochromic Sensor, and ML-random forest model (Table 2).
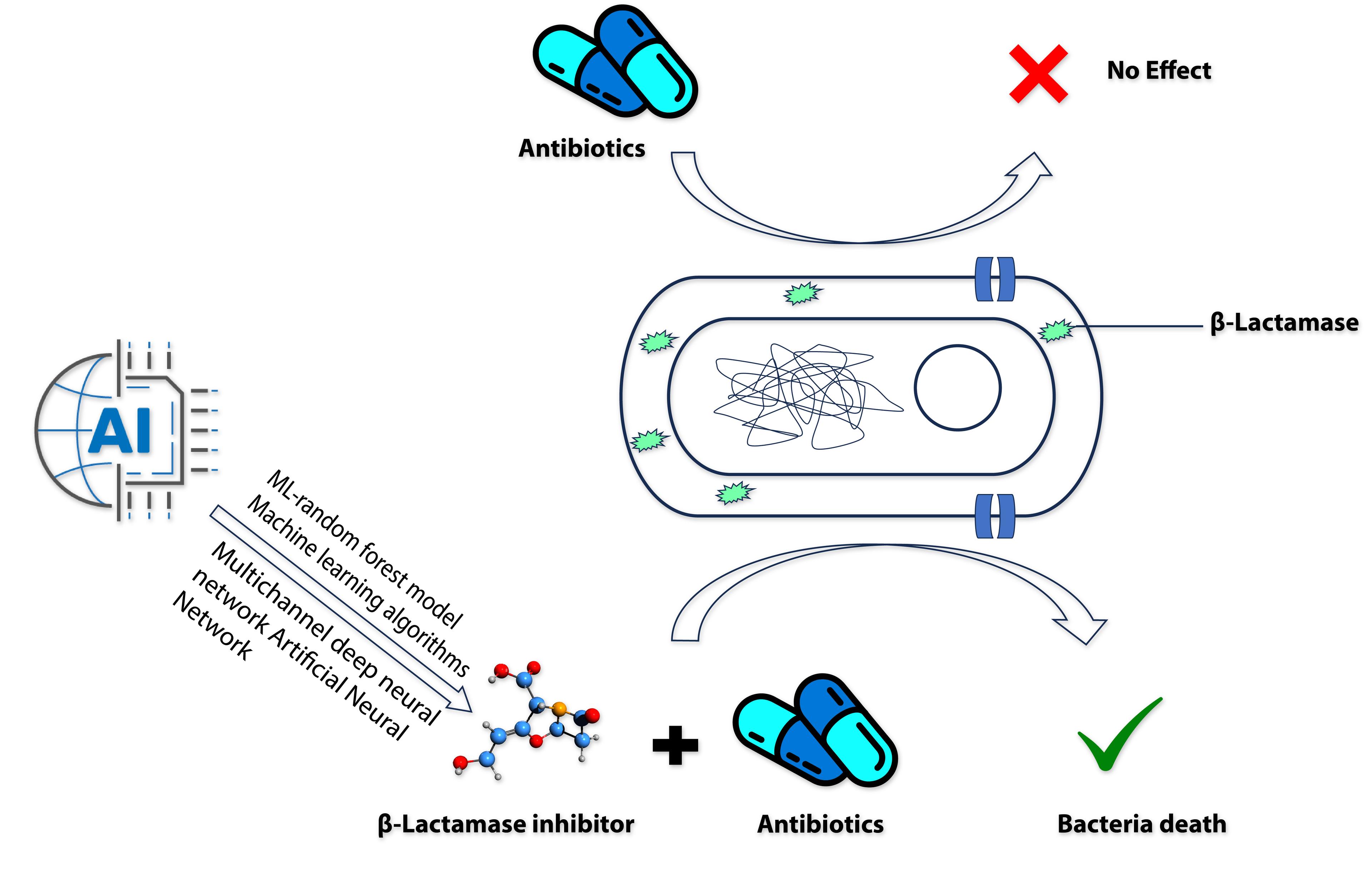
Table 2. Recent AI technologies are used to develop and identify beta-β-lactamase inhibitors.
AI TO IDENTIFY AMR MARKERS
AMR markers are specific traits or genetic components linked to antibiotic resistance of bacteria or other microorganisms. These markers are used in diagnostic tests to identify and detect the presence of AMR in pathogens. The value of AMR markers is found in their ability to direct appropriate treatment choices. Determining the resistance markers present in the pathogen when a patient is infected with a bacterium resistant to a particular antimicrobial drug is critical. This knowledge aids medical professionals in selecting the most appropriate antibiotics to treat the infection. The likelihood of a successful outcome can be increased while reducing the chance of further fostering AMR by choosing the best antibiotics.
The genes in charge can be found in various ways, and researchers are still developing new technology. The most popular techniques for identification are based primarily on cultivating these microorganisms under particular circumstances. Though straightforward and simple to implement, some aspects could be more beneficial. Some research projects are hampered by the existence of viable, non-cultivable microorganisms or by the lengthy time it takes for some organisms to multiply in the environment [71].
In recent years, AI-based machine learning and deep learning methods (Figure 3) have demonstrated substantial performance in AMR control, such as sequencing-based AI applications, gathering clinical data to develop clinical decision support systems [72]. Different AI algorithms are widely used for AMR detection, including naïve Bayes (NB), support vector machines (SVM), decision trees (DT), random forests (RF), and artificial neural networks (ANN) [73]. The technique has also been successfully used to detect functions and abnormalities of heart, kidney, liver, thyroid, lung, gastrointestinal etc. [68].
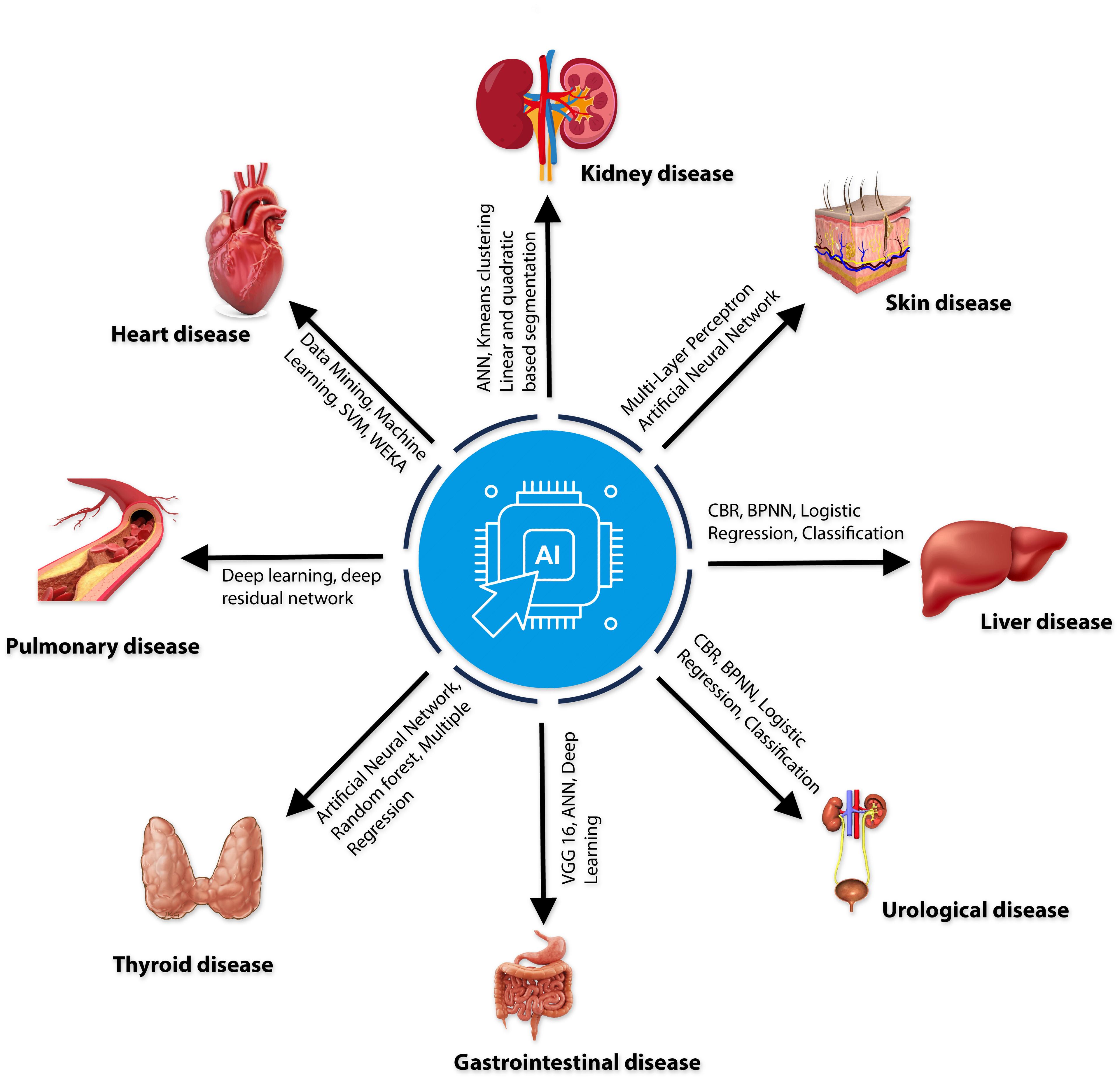
It is necessary to detect AMR markers to fight AMR and provide accurate treatment to the patient. Recent studies have shown that deep learning, a class of machine learning algorithms, can also expand the catalogue of AMR genes and increase the accuracy of the predictions based on metagenomic data. Recent studies have shown that the deep learning methodology, known as DeepARG, is more accurate than more conventional methods when identifying antibiotic resistance genes based on metagenomic data [75].
Various AI-based machine learning and deep learning methods successfully identify the resistance genes from different bacteria. Machine Learning, Homology Modeling, and Molecular Docking were used to detect the specific gene and effect of particular antibiotics on many bacterial with 90% accuracy [76]. Models such as deep convolutional neural network (DCNN) and support vector machine (SVM) has been used to detect specific gene identification of tuberculosis with a accuracy of about 93% accuracy [77]. The DNP-AAP (deep neural pursuit - average activation potential) method was used recently to analyse the Neisseria gonorrhoeae dataset with paired whole-genome sequence data and resistance profiles to five commonly used antibiotics.
AI TO COMBAT BIOFILM FORMATION
Biofilm is a pivotal cause of AMR. It is a colony of heterogeneous, complex matrix structures where bacteria and organisms play some part role. It differs from their planktonic structure and is highly resistant to antimicrobial properties compared with planktonic characteristics [78]. This matrix is formed by exopolysaccharides, extracellular DNA(eDNA), proteins, and lipids where bacteria and other organisms attach deeply to adhesive materials [79, 80]. For this tangled form of the matrix, deeply residing organisms can easily survive in harsh conditions and perform antimicrobial inhibitory action combined with several factors, which are the number of organisms, quorum sensing (QS), mutation, longevity of cells, upregulation of drug efflux pumps, and Antibiotic tolerance. So, it is difficult to treat the infection for organisms associated with biofilm. Therefore, it's essential to identify the mechanism and structure of biofilm to mitigate AMR. However, in mechanism, organisms come close and Attach to the surface, secrete matrix and adhesive materials, then mature the matrix and disperse into the environment. To prevent biofilm development, it is also essential to understand the structure of biofilm. Understanding biofilm can be achieved in qualitative and quantitative ways. If biofilm detection can be done, it will help to reduce AMR.
Identification of biofilm formation followed by biofilm inhibitory ways is very important. Detection of biofilm and inhibitory molecules usually requires more time and is arduous. So, it’s essential to identify as early as possible. There are many types of methods to combat biofilm such are Inhibition of macromolecule synthesis and adhesion of cells, Anti-biofilm peptides, Bacteriophage (phage) therapy, Antimicrobial Photodynamic Therapy, alteration of membrane permeabilization, inhibition of cell division or cell survival, antibiotic-modifying enzymes in the matrix, Nutritional limitation and stress response, Electrochemical Control of Biofilm Formation, pH-Responsive Polymer–Drug Conjugate system and nanotechnology. In recent research, combined with previous studies, machine learning and some other AI models have brought outstanding achievements in detecting biofilm inhibitory molecules, which is also a less time-consuming task (Table 3).
aBiofilm is a further tool for chemical molecule anti-biofilm prediction that offers a qualitative prediction of molecule inhibitory action. The aBiofilm tool makes use of a quantitative structure-activity relationships (QSAR) model that is based on Support Vector Machine (SVM), and it assigns low (negative) and high (positive) labels to tiny molecules by their relative inhibitory potentials of 20 and 60 %, respectively [81]. One of its flaws is that it needs to consider scenarios where model-building inhibition is between 20 and 60%, which might lead to errors in prediction and reduce its effectiveness [82].
Table 3. Different methods of formation and detection of biofilm.
AI AND ANTIBIOTIC SUSCEPTIBILITY TEST
Antibiotics are a life-saving medicine for chronic infectious diseases where Infections caused by bacteria are prevented and treated using it. Despite the beneficial effects of an antibiotic in treating infectious diseases, it can also cause some life-threatening impacts on human and animal life due to overuse or misuse by AMR.
There are several convenient ways to conduct an antibiotic sensitivity test (AST), but it’s usually time-consuming and takes about 16-24 hours. There are usually two types of AST: the phenotypic and genotypic tests. In phenotypic AST, Disk Diffusion Gradient Diffusion, Beta-β-lactamase Activity, Broth dilution, Agar dilution, Epsilometer testing (EST), Matrix-Assisted Laser Desorption lionization-Time of flight mass spectrometry (MALDI-TOF MS), Flow cytometry are done. PCR, DNA microarrays, DNA chips, and loop-mediated isothermal amplification (LAMP) are frequently employed in genotypic procedures. However, new AI technology has been developed recently to perform AST rapidly. Support Vector Machines, Genetic Methods, Artificial Neural Networks, and Quick Classifiers are popular AI techniques. AST usually uses these algorithms for machine learning.
In another study, Antibiotic effectiveness in treating different bacteria kinds was predicted using an ANN model, which was then confirmed. The study considered susceptibility as the output, with organism name, specimen type, and antibiotic name as inputs. To achieve this, a model with a single input layer, one hidden layer, and one output layer topology was developed and trained using data from the Queensland government's website. The study shows that the proposed ANN model utilizing the JNN tool has a 94.17% accuracy rate in predicting an organism's susceptibility to antibiotics [96]. In this study results varied from 60% to 100 % [97]. For rapid result of AST report, here MALDI-TOF-based medical AI (XBugHunter) was used successfully with an accuracy above 95%. Therefore, the application of XBugHunter might be able to lower the over-prescription of antibiotics in patients with S. aureus bacteremia [98].
CONCLUSION AND RECOMMENDATION
Early and accurate detection of AMR of pathogens is crucial to prescribe effective drugs for therapeutic purposes. In this review, we tried to focus on how AI is used nowadays, along with other techniques in AMR detection. We found that integrating AI in AMR detection has proven to be a transformative step in combating this global health threat. AI technologies have led to significant advancements in the rapid and accurate identification of AMR pathogens, enabling healthcare professionals to make informed decisions regarding patient treatment and containment measures. Consequently, the use of AI in AMR detection has contributed to reducing AMR risk, safeguarding public health, and promoting responsible antibiotic usage. One of the critical strengths of AI in AMR detection lies in its ability to analyze vast amounts of data from diverse sources, including genomic sequences, patient records, and real-time epidemiological data. This capability has facilitated the early identification of emerging AMR strains, allowing for prompt and targeted interventions to prevent their spread. Moreover, AI-powered diagnostic tools have enhanced the accuracy of antimicrobial susceptibility testing, streamlined treatment selection, and minimized the misuse of antibiotics.
Despite AI's benefits in detecting AMR, it still has significant drawbacks. First, accuracy is lower because there is a lack of data, and it is challenging for existing AI algorithms to forecast high-dimensional traits. Therefore, an area for future research is the automatic annotation of unlabeled data using unsupervised learning. Second, the generalizability of AI-based systems is insufficient because they can only handle datasets with the same distribution. Transfer learning and few-shot learning will, therefore, be used more and more in the future to counteract AMR. Finally, applying AI technology quickly is challenging due to the severe lack of infrastructure, the high installation cost, data authenticity, data normalization, and the shortage of skilled labor.
To strengthen AI's involvement in AMR detection and handling related issues in the healthcare industry, we recommend the following areas to be explored in future research in this field:
Enhance predictive models: Develop a more sophisticated model that includes multiple parameters, such as genomic information, patient history, and environmental factors, to predict AMR more accurately and effectively.
Data sharing and standardization: Encourage international collaboration and data sharing among healthcare institutions and researchers to standardize data formats and interoperability protocols that will ultimately improve the accuracy and robustness of AI models, enabling better generalization across different populations and settings.
Ethical and regulatory frameworks: Develop and implement clear ethical guidelines and regulatory frameworks for using AI in AMR detection. This includes ensuring data privacy and security, mitigating algorithm bias, and promoting transparency in AI decision-making processes.
Building capacity: Invest in effectively training healthcare professionals and researchers to use AI tools for AMR detection. Building capacity at all healthcare system levels will enable a seamless integration of AI technologies into routine clinical practice.
Real-time surveillance: Strengthen real-time surveillance systems that integrate AI algorithms to monitor the emergence and spread of AMR. Early detection of resistant strains is crucial for implementing timely containment measures and preventing outbreaks.
One health approach: Adopt a holistic One Health approach that considers human, animal, and environmental factors in AMR surveillance. AI may help analyze data from multiple sources to better comprehend the intricate interactions between these aspects.
Investment in research and development: The authorities should take this matter seriously because AMR is a national and worldwide concern, so research connected to AMR and AI should be expanded with sufficient government and drug industry funding.
AMR is a global health problem having negative impact on humans, animals, and the ecosystem. We can strengthen our group efforts to reduce AMR by embracing these suggestions and developing AI integration in AMR detection. To maintain the effectiveness of current antimicrobial agents and protect the health of future generations, it will be essential to combine the power of AI with efficient public health initiatives and responsible antibiotic stewardship. By working together, we can defeat AMR and pave the road for a better and healthier future.
ACKNOWLEDGEMENT
A special thanks to Samina Ievy for valuable comments and suggestions on preparing this review.
AUTHOR CONTRIBUTIONS
MTR conceived the idea. MTR, RIM, and NAIF prepared the outline of the review. RIM and NAIF performed the literature search and data extraction, analysis of extracted data and manuscript preparation. MSI and MLR also contributed to manuscript preparation and editing. MTR supervised the manuscript preparation. MTR, RIM, NAIF and MLR prepared the final draft. All authors read and accepted the final version of the manuscript.
CONFLICTS OF INTEREST
There is no conflict of interest among the authors.
References
- [1]Watkins RR, Bonomo RAJIDC. Overview: Global and local impact of antibiotic resistance. 2016;30:313-22.
- [2]Cummins J. Antimicrobial resistance. The New Zealand medical journal. 1999;112:166-7.
- [3]UNEP. Antimicrobial resistance: A global threat. UN Environment Programme; 2016.
- [4]CDC. Antibiotic resistance threats in the united states. CDC; 2021.
- [5]Martínez JL, Coque TM, et al. What is a resistance gene? Ranking risk in resistomes. 2015;13:116-23.
- [6]Prestinaci F, Pezzotti P, et al. Antimicrobial resistance: A global multifaceted phenomenon. 2015;109:309-18.
- [7]Dever LA, Dermody TSJAoim. Mechanisms of bacterial resistance to antibiotics. 1991;151:886-95.
- [8]Mansur A, Saleem Z, et al. Role of artificial intelligence in risk prediction, prognostication, and therapy response assessment in colorectal cancer: Current state and future directions. 2023;13:1065402.
- [9]Tran NK, Kretsch C, et al. Machine learning and artificial intelligence for the diagnosis of infectious diseases in immunocompromised patients. 2023;36:235-42.
- [10]Paul D, Sanap G, et al. Artificial intelligence in drug discovery and development. 2021;26:80.
- [11]Melo MC, Maasch JR, et al. Accelerating antibiotic discovery through artificial intelligence. 2021;4:1050.
- [12]Kauffman HJARiAI, Computing C. Artificial intelligence and public health: A descriptive review of use cases. 2022;5:29-37.
- [13]Dadgostar PJI, resistance d. Antimicrobial resistance: Implications and costs. 2019:3903-10.
- [14]Llor C, Bjerrum LJTaids. Antimicrobial resistance: Risk associated with antibiotic overuse and initiatives to reduce the problem. 2014;5:229-41.
- [15]Jernigan JA, Hatfield KM, et al. Multidrug-resistant bacterial infections in us hospitalized patients, 2012–2017. 2020;382:1309-19.
- [16]Murray CJ, Ikuta KS, et al. Global burden of bacterial antimicrobial resistance in 2019: A systematic analysis. 2022;399:629-55.
- [17]Van Hauwermeiren E, Iosifidis E, et al. Development of case vignettes for assessment of the inter-rater variability of national validation teams for the point prevalence survey of healthcare-associated infections and antimicrobial use in european acute care hospitals. 2019;101:455-60.
- [18]Taneja N, Sharma MJTIjomr. Antimicrobial resistance in the environment: The indian scenario. 2019;149:119.
- [19]Tazerji SS, Nardini R, et al. An overview of anthropogenic actions as drivers for emerging and re-emerging zoonotic diseases. 2022;11:1376.
- [20]Morgan DJ, Okeke IN, et al. Non-prescription antimicrobial use worldwide: A systematic review. 2011;11:692-701.
- [21]Hassell JM, Begon M, et al. Urbanization and disease emergence: Dynamics at the wildlife–livestock–human interface. 2017;32:55-67.
- [22]McEwen SA, Fedorka-Cray PJJCid. Antimicrobial use and resistance in animals. 2002;34:S93-S106.
- [23]Gelband H, Miller-Petrie, M., Pant, S., Gandra, S., Levinson, J., Barter, D., . The state of the world’s antibiotics. Washington, dc. Centre for Disease Dynamics, Economics & Policy; 2015.
- [24]US-FDA. Antibiotic resistance threats in the united states. CDC; 2019.
- [25]Van Boeckel TP, Brower C, et al. Global trends in antimicrobial use in food animals. 2015;112:5649-54.
- [26]Padungton P, Kaneene JBJJoVMS. Campylobacter spp. In human, chickens, pigs and their antimicrobial resistance. 2003;65:161-70.
- [27]Alfredson DA, Korolik VJFml. Antibiotic resistance and resistance mechanisms in campylobacter jejuni and campylobacter coli. 2007;277:123-32.
- [28]Jaja IF, Bhembe NL, et al. Molecular characterisation of antibiotic-resistant salmonella enterica isolates recovered from meat in south africa. 2019;190:129-36.
- [29]UNEP. Antimicrobial resistance: A global threa. Unep; 2016.
- [30]Hazards EPoB, Koutsoumanis K, et al. Role played by the environment in the emergence and spread of antimicrobial resistance (amr) through the food chain. 2021;19:e06651.
- [31]McClellan K, Halden RUJWr. Pharmaceuticals and personal care products in archived us biosolids from the 2001 epa national sewage sludge survey. 2010;44:658-68.
- [32]Zhang Q-Q, Ying G-G, et al. Comprehensive evaluation of antibiotics emission and fate in the river basins of china: Source analysis, multimedia modeling, and linkage to bacterial resistance. 2015;49:6772-82.
- [33]Akiba M, Senba H, et al. Impact of wastewater from different sources on the prevalence of antimicrobial-resistant escherichia coli in sewage treatment plants in south india. 2015;115:203-8.
- [34]Masud MRI, Suman KH, et al. A review on enhanced microplastics derived from biomedical waste during the covid-19 pandemic with its toxicity, health risks, and biomarkers. 2022:114434.
- [35]Verweij PE, Snelders E, et al. Azole resistance in aspergillus fumigatus: A side-effect of environmental fungicide use? 2009;9:789-95.
- [36]Ahmad M, Vithanage M, et al. Inhibitory effect of veterinary antibiotics on denitrification in groundwater: A microcosm approach. 2014;2014.
- [37]Turing AM. Computing machinery and intelligence: Springer; 2009.
- [38]Monett D, Lewis C, et al. Introduction to the jagi special issue ‘on defining artificial intelligence’–commentaries and author’s response. 2020;11:1-100.
- [39]Kok J, Boers E, et al. Knowledge for sustainable development: An insight into the encyclopedia of life support systems, Artificial intelligence: Definition, trends, techniques and cases. 2010:1096-7.
- [40]Schuett JJL, Innovation, Technology. Defining the scope of ai regulations. 2023;15:60-82.
- [41]Liu F, Zhou Z, et al. Deep learning approach for evaluating knee mr images: Achieving high diagnostic performance for cartilage lesion detection. 2018;289:160-9.
- [42]Wahl B, Cossy-Gantner A, et al. Artificial intelligence (ai) and global health: How can ai contribute to health in resource-poor settings? 2018;3:e000798.
- [43]Comito C, Falcone D, et al. Current trends and practices in smart health monitoring and clinical decision support. 2020 IEEE International Conference on Bioinformatics and Biomedicine (BIBM): IEEE; 2020. p. 2577-84.
- [44]Klevens RM, Edwards JR, et al. Changes in the epidemiology of methicillin-resistant staphylococcus aureus in intensive care units in us hospitals, 1992–2003. 2006;42:389-91.
- [45]Saleh N, Awada S, et al. Evaluation of antibiotic prescription in the lebanese community: A pilot study. 2015;5:27094.
- [46]Jawaid A, Hafeez J, et al. Lung cancer detection using artificial neural network on android. 2023 Global Conference on Wireless and Optical Technologies (GCWOT): IEEE; 2023. p. 1-8.
- [47]Chen J, Remulla D, et al. Current status of artificial intelligence applications in urology and their potential to influence clinical practice. 2019;124:567-77.
- [48]Khan MA, Khan MA, et al. Gastrointestinal diseases segmentation and classification based on duo-deep architectures. 2020;131:193-204.
- [49]Gouda W, Yasin RJEJoR, et al. Covid-19 disease: Ct pneumonia analysis prototype by using artificial intelligence, predicting the disease severity. 2020;51:1-11.
- [50]Vasal S, Jain S, et al. Covid-ai: An artificial intelligence system to diagnose covid 19 disease. 2020;9:1-6.
- [51]Ostovar A, Ehsani-Chimeh E, et al. The diagnostic value of ct scans in the process of diagnosing covid 19 in medical centers. 2020.
- [52]Gao XW, James-Reynolds C, et al. Analysis of tuberculosis severity levels from ct pulmonary images based on enhanced residual deep learning architecture. 2020;392:233-44.
- [53]Das DK, Mukherjee R, et al. Computational microscopic imaging for malaria parasite detection: A systematic review. 2015;260:1-19.
- [54]Kylberg G, Uppström M, et al. Segmentation of virus particle candidates in transmission electron microscopy images. 2012;245:140-7.
- [55]Hay EA, Parthasarathy RJPcb. Performance of convolutional neural networks for identification of bacteria in 3d microscopy datasets. 2018;14:e1006628.
- [56]Li S, Zhang YJMDV. Microscopic images of parasites species. 2020.
- [57]Zieliński B, Sroka-Oleksiak A, et al. Deep learning approach to describe and classify fungi microscopic images. 2020;15:e0234806.
- [58]Coleman KJCoim. Diazabicyclooctanes (dbos): A potent new class of non-β-lactam β-lactamase inhibitors. 2011;14:550-5.
- [59]Reygaert WCJAm. An overview of the antimicrobial resistance mechanisms of bacteria. 2018;4:482.
- [60]Bush K, Jacoby GAJAa, et al. Updated functional classification of β-lactamases. 2010;54:969-76.
- [61]Poirel L, Nordmann PJCM, et al. Carbapenem resistance in acinetobacter baumannii: Mechanisms and epidemiology. 2006;12:826-36.
- [62]Parvaiz N, Ahmad F, et al. Discovery of beta-lactamase cmy-10 inhibitors for combination therapy against multi-drug resistant enterobacteriaceae. 2021;16:e0244967.
- [63]Dong R, Yang H, et al. Deepbli: A transferable multichannel model for detecting β-lactamase-inhibitor interaction. 2022;62:5830-40.
- [64]Anant PS, Gupta P. Application of machine learning in understanding bioactivity of beta-lactamase ampc. Journal of Physics: Conference Series: IOP Publishing; 2022. p. 012005.
- [65]Wang Y, Li F, et al. Deepbl: A deep learning-based approach for in silico discovery of beta-lactamases. 2021;22:bbaa301.
- [66]Tan X, Tang Y, et al. Explainable deep learning-assisted photochromic sensor for β-lactam antibiotic identification. 2023;95:3309-16.
- [67]Skvortsova A, Trelin A, et al. Sers and advanced chemometrics–utilization of siamese neural network for picomolar identification of beta-lactam antibiotics resistance gene fragment. 2022;1192:339373.
- [68]Chen J, Liu Y, et al. Combined support-vector-machine-based virtual screening and docking method for the discovery of imp-1 metallo-β-lactamase inhibitors supplementary data. 2013;1:120-31.
- [69]Yu T, Fu Y, et al. Identification of antibiotic resistance in eskape pathogens through plasmonic nanosensors and machine learning. 2023;17:4551-63.
- [70]Ashraf MA, Khan YD, et al. Βlact-pred: A predictor developed for identification of beta-lactamases using statistical moments and pseaac via 5-step rule. 2021;2021.
- [71]Braga PAC, Tata A, et al. Bacterial identification: From the agar plate to the mass spectrometer. 2013;3:994-1008.
- [72]Chowdhury AS, Lofgren ET, et al. Identifying predictors of antimicrobial exposure in hospitalized patients using a machine learning approach. 2020;128:688-96.
- [73]Lv J, Deng S, et al. A review of artificial intelligence applications for antimicrobial resistance. 2021;3:22-31.
- [74]Kumar Y, Koul A, et al. Artificial intelligence in disease diagnosis: A systematic literature review, synthesizing framework and future research agenda. 2022:1-28.
- [75]Arango-Argoty G, Garner E, et al. Deeparg: A deep learning approach for predicting antibiotic resistance genes from metagenomic data. 2018;6:1-15.
- [76]Sunuwar J, Azad RKJM. Identification of novel antimicrobial resistance genes using machine learning, homology modeling, and molecular docking. 2022;10:2102.
- [77]Oloko-Oba M, Viriri S. Diagnosing tuberculosis using deep convolutional neural network. Image and Signal Processing: 9th International Conference, ICISP 2020, Marrakesh, Morocco, June 4–6, 2020, Proceedings 9: Springer; 2020. p. 151-61.
- [78]Stewart PS, Costerton JWJTl. Antibiotic resistance of bacteria in biofilms. 2001;358:135-8.
- [79]Flemming H-C, Wingender JJNrm. The biofilm matrix. 2010;8:623-33.
- [80]Hall CW, Mah T-FJFmr. Molecular mechanisms of biofilm-based antibiotic resistance and tolerance in pathogenic bacteria. 2017;41:276-301.
- [81]Rajput A, Thakur A, et al. Abiofilm: A resource of anti-biofilm agents and their potential implications in targeting antibiotic drug resistance. 2018;46:D894-D900.
- [82]Srivastava GN, Malwe AS, et al. Molib: A machine learning based classification tool for the prediction of biofilm inhibitory molecules. 2020;112:2823-32.
- [83]Olivares E, Badel-Berchoux S, et al. The biofilm ring test: A rapid method for routine analysis of pseudomonas aeruginosa biofilm formation kinetics. 2016;54:657-61.
- [84]Tasse J, Cara A, et al. A steam-based method to investigate biofilm. 2018;8:13040.
- [85]Kırmusaoğlu SJA, antibiotic resistance, antibiofilm strategies, methods a. The methods for detection of biofilm and screening antibiofilm activity of agents. 2019;7.
- [86]Freeman D, Falkiner F, et al. New method for detecting slime production by coagulase negative staphylococci. 1989;42:872-4.
- [87]Christensen GD, Simpson WA, et al. Methods for evaluating attached bacteria and biofilms: An overview. 2000:213-33.
- [88]Ragi S, Rahman MH, et al. Artificial intelligence-driven image analysis of bacterial cells and biofilms. 2021.
- [89]Ramos-Martinez E, Herrera M, et al. A multi-disciplinary procedure to ascertain biofilm formation in drinking water pipes. 2016.
- [90]Vyas N, Sammons R, et al. A quantitative method to measure biofilm removal efficiency from complex biomaterial surfaces using sem and image analysis. 2016;6:32694.
- [91]Buetti-Dinh A, Galli V, et al. Deep neural networks outperform human expert's capacity in characterizing bioleaching bacterial biofilm composition. 2019;22:e00321.
- [92]Zhang RY, Zhang YT, et al. Initial attachment and biofilm formation of a novel crenarchaeote on mineral sulfides. 2015;1130:127-30.
- [93]Gierl L, Stoy K, et al. An open-source robotic platform that enables automated monitoring of replicate biofilm cultivations using optical coherence tomography. 2020;6:18.
- [94]Hartmann R, Jeckel H, et al. Quantitative image analysis of microbial communities with biofilmq. 2021;6:151-6.
- [95]Futo M, Široki T, et al. A novel time-lapse imaging method for studying developing bacterial biofilms. 2022;12:21120.
- [96]Oriban AJA, Abdel-Al SN, et al. Antibiotic susceptibility prediction using jnn. 2020;4.
- [97]Weis CV, Jutzeler CR, et al. Machine learning for microbial identification and antimicrobial susceptibility testing on maldi-tof mass spectra: A systematic review. 2020;26:1310-7.
- [98]Wang H-Y, Chung C-R, et al. Extensive validation and prospective observation of the impact of an ai-based rapid antibiotics susceptibility prediction platform in multiple medical centers. 2021:2021.06. 23.21259391.